E-News August 2015 Edition
Hybrid Control Systems for New Challenges by Professor Mike J Grimble

There are many areas of advanced control theory which are rather abstract and remote from real applications. In some cases it takes a long time before such methods are sufficiently mature an understandable to be used in industry. Hybrid systems control is one of those topics. By this of course I do not mean hybrid vehicles but instead the control of systems that are very mixtures of continuous and possible switching behaviour.
One of the most important aspects of process control systems is the safety logic and interlocks that ensure mistakes cannot be made nor do automoati9c systems malfunction to cause dangerous chemical reactions. In years gone by these would of course be set up through relay logic but this was superseded by PLC's and Scada systems. This discrete decision making and switching behaviour is rather different to the continuous cooperation of a process plant where for example liquid level is controlled where the physical process is continuous.
Switching is of course often involved in industrial processes and other applications. This can result in abrupt changes in behaviour so the output of the system has periods of continuous change followed by an instantaneous abrupt change. Changing gears in an automobile is one example of where the behaviour completely changed over a very short time.
There are therefore a very large number of situations where a combination of continuous and what might be termed discreet event behaviour occurs. It was therefore recognised long ago that a theory should be produced, termed hybrid control, to provide a comprehensive and unified solution to such problems.
As with most control engineers design work often starts with system modelling and for hybrid systems there were many options. One of the earliest models to be used was the piecewise linear model. Nonlinear systems are of course often approximated by linear models around given operating points. The piecewise linear approach to hybrid systems modelling therefore covers nonlinear systems and can represent the abrupt change in behaviour which may arise.
A wider class of hybrid systems is termed linear complementarity systems. The states in such systems can represent groups of continuous states and those representing the discreet events. Since ma y of the problems in hybrid control result from logical decision making combined with continuous operation it is natural to replace Boolean algebra processes of true and false by the binary integers 0 and 1. Superficially the equations of a combined system in say linear complementarity form looks similar to regular system equations with the addition of an algebraic equation representing constraints.
Another type of hybrid system model is the mix of logical dynamic model which has become popular for use in control systems design. All of these models are related but some are more general and more convenient to use in particular hybrid problems.
Further details can be obtained from Professor Mike Grimble
Mike Grimble
Advanced Control Systems, 22nd - 25th September 2015, Glasgow
This four day training course will introduce control systems design techniques for applications that are severely nonlinear, requiring control that is more advanced than classical PID. Computer-based exercises are used throughout so that attendees get hands-on experience with the methods. Please note that individual days can be selected, should you not need every topic covered.
The course will start by covering Kalman Filter algorithms and their implementation as an observer and parameter estimation, as advanced control often relies on plant models and state estimates. The Extended Kalman Filter is specifically useful for nonlinear systems and their control.
This will be followed by an overview of popular industrial techniques for nonlinear control systems design, and will introduce a new family of nonlinear industrial controllers referred to as Nonlinear Generalised Minimum Variance (NGMV). These new NGMV controllers are simple to derive theoretically and easy to implement, and can extend to include optimal and predictive features.
The third day will cover model predictive control (MPC) which the most popular advanced control technique adopted by the industry. Its popularity stems from the capability to handle constraints and an optimal control framework. The extension to nonlinear MPC will then be presented, including some new methods with simpler implementation than other approaches such as on-line linearisation or adaption. Application examples will be used to illustrate the various methods. A brief overview on the current trends in Nonlinear Predictive Control will conclude the course.
Agenda and online registration is avaliable here or further details may be obtained from Dr Meghan McGookin
Developments in Smith Predictor Control
The Smith Predictor is used in many application areas for systems which have long transport delays in comparison with dominant plant time constants. It was developed by Professor O.J. M Smith at Berkeley, and first came to popularity in the process industries. However, it is now also used in automotive, servo system and metal processing applications. Peoples experience with Smith Predictors depends very much on the application and can range from "they work very well" or "they only work when the plant models are good".
The problems with Smith Predictors may be listed as:
The Smith Predictor has a structure involving an ideal feedback loop without the transport delay term and then a parallel path with the plant model which generates an estimate of the disturbance.
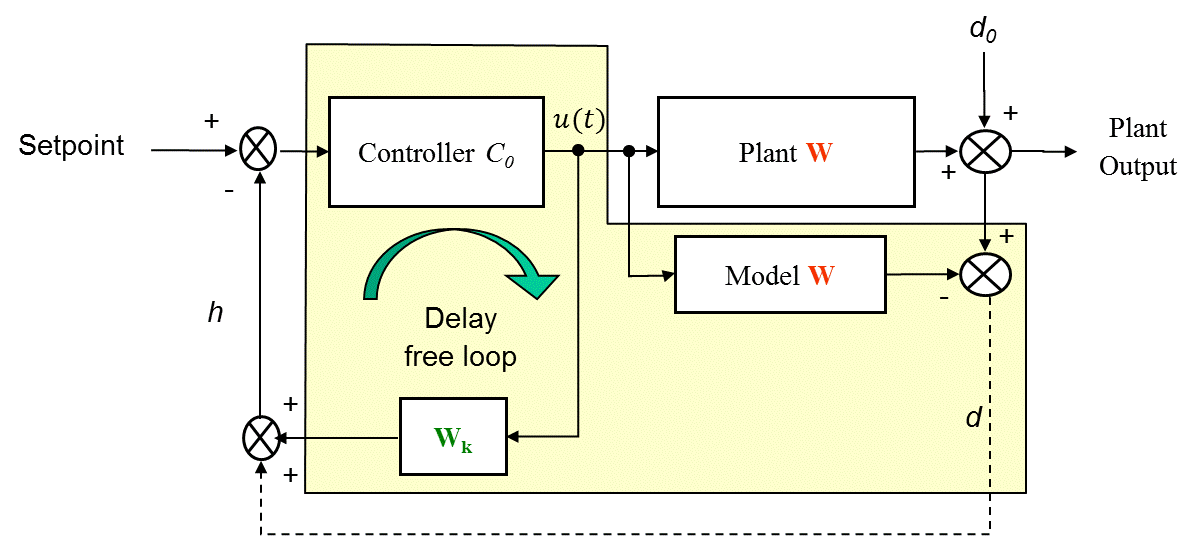
Some of the above questions arise because the Smith Predictor is simply a structure to cope with the transport delay term, but it gives no guidance whatsoever on how to design the feedback controller block C0. The controller within the delay free loop must be selected by the designer and might for example be in PID or lead lag controller. Knowledge of the Smith structure gives no information on how to design this controller and therefore there is no help in the problems of disturbance rejection, multivariable interactions, or even with a nonlinearities in the plant. The conclusion is that the control designer must use existing classical skills or advanced techniques to design the ideal (delay free) feedback loop controller. This task is of course simplified because it does not contain the transport delay element.
Advanced control methods provide tools to tackle each of these problems whilst still maintaining a simple structure. For example, restricted structure optimal control enables a PID type structure to be specified where the gains are chosen to minimise and LQG or an H? cost index. The effects of uncertainties can also be compensated once a structure for the uncertainties has been determined. However, engineers that use the Smith Predictor are more likely to want to use classical type methods to deal with these design issues. Each of the problems will therefore be considered in classical control design terms.
The problems concerning modelling errors and uncertainties are probably the most difficult. The parallel path in the Smith Predictor that involves the plant in one path and the model of the plant in the other, the outputs of which are differenced, is the main culprit for problems. When there is mismatch the situation is similar to having another feedback loop around the ideal (delay free) subsystem. Since in the design it is assumed that this does not contain the transport delay of the system the result is destabilizing.
The modelling errors may be due to either the difference in the dynamics of the plant model, or due to the error in knowledge of the transport delay. In fact a common problem is when the transport delay in the plant is actually time-varying. This for example occurs when using temperature control in a steel mill where the line speed is of course varying and the transport delay therefore changes. An effort can of course be made to remove the uncertainty, which in the steel mill case would involve changing the model for the delay within the Smith Predictor online, and this is certainly feasible and relatively simple.
If there is knowledge that the model mismatch is in a particular frequency range then it may be possible to introduce a filter on the disturbance estimate, with low gain in the region of the uncertainty. This of course becomes a problem if the disturbance itself is active in this frequency range. The knowledge of the disturbance is important if good disturbance rejection is required and hence the use of filtering will require trade-offs to be made.
The other tuning variable that can be used is the choice of ideal feedback loop controller C0. This effects the sensitivity functions for an ideal system without transport delay. These are not the same as the actual sensitivities which arise when there is model mismatch (due to the additional feedback term introduced). However, a system with poor ideal loop sensitivities is unlikely to be robust when there is model mismatch presence. This suggests careful design of the ideal feedback loop controller will be beneficial.
One of the major problems with the Smith Predictor that illustrates the sensitivity problems that can arise is that it cannot stabilise open-loop unstable systems. This is due to the parallel path between the plant and the plant model which effectively hides any unstable modes, and leaves the system unstable whatever action is taken in the ideal feedback loop. It follows that if the plant model is close to being unstable difficulties are likely to arise and a common case is when an integrator is present. The plant model is then critically stable and model mismatch can result in instability. The reason the Smith Predictor works well in many process plants is that the plant model often involves a simple time constant and a transport delay term. The real dynamics of the process are probably very much more complex than this, but the model approximation can often be very good.
To achieve good disturbance rejection is usually easier, and can be achieved through the design of the ideal feedback loop controller C0. Since most systems have disturbances to be attenuated at low frequencies it requires the controller gain to be high at low frequencies. Model mismatch will cause an error but this is not usually significant in such a case.
If the system is multivariable with significant interactions simple methods like decoupling may be applied. If the plant is non-square this complicates the classical designs and the use of more advanced methods may be easier.
For the attenuation of measurement noise the ideal feedback loop controller should just have good roll off at high frequencies. To cope with the nonlinearities the model of the plant, in the Smith Predictor, can be augmented to include the nonlinear elements. However, the subsequent controller is of course then nonlinear. If a simpler solution is needed then the Smith Predictor can be computed for a number of linearized models and then the controller gains within the ideal feedback loop can be switched according to operating region.
The Smith Predictor structure is related to the so called internal model control structures and it is similar to the Q Parametrisation used by Professor John Moore at the Australian National University in Canberra. Closer to home, it is related to the nonlinear generalised minimum variance controller, which is described on ACTC training courses, for both linear and nonlinear systems. For difficult systems there are therefore a number of advanced control transport delay compensation techniques which can be used, including those with adaptive features.
Even though the Smith Predictor has many design problems there are some applications where it works relatively easily, with little fuss, and without needing a great depth of knowledge on advanced control. Transport delays in systems can often cause significant difficulties with controller tuning, and the Smith Predictor can provide simple and intuitive method of compensating for such delays. It is recommended for inclusion in the toolkit of all practical application control engineers.
Applications News
Ship Positioning Systems
Recent advances on ship positioning systems control enables the nonlinearities in the systems to be dealt with more accurately, with the potential for position improvements across the range of operation.
Machinery Controls
Up until very recently high speed machinery control could not utilise nonlinear predictive control methods because of their complexity. However, new and more practical nonlinear predictive algorithms are now available which should be particularly valuable for high accuracy, high speed servo systems.
Steel Mill Controls
New approaches to the tuning of controllers for steel mill controls and for novel condition monitoring/benchmarking have been funded by the European Union in recent cooperative projects between the University of Strathclyde and ISC.
Automotive Controls
The number of control loops continues to grow in modern automotive systems and there are new problems arising in the control of driverless vehicles. A common feature is the need for controllers which are economical to implement in the electronic control units available. The Matlab based advanced control toolbox ISC has developed has a range of controller types specifically designed for this type of application. A new springer book will be published later this year providing design methods and describing applications.
The Flexibility of Kalman Filters
The Kalman filter plays a very important role as part of the LQG feedback controller. However, it is also important in pure estimation problems like the pooling of measurements from different sources to get high accuracy estimates of position. The Kalman filter and the related family of linear observers are also used extensively in fault monitoring and detection systems. It is therefore one of the most valuable assets to the control engineer, but also to the signal processing, and even communications engineer.
A question is how general is the Kalman filter, since it was designed for linear systems. It is of course available in continuous-time and discrete-time versions and the system model can be time invariant or can be time-varying. In this latter case the state-space matrices can have time varying parameters. It can also be used on steady-state problems or on short time scale estimation problems. That is, where the system has been switched on for a long time and has therefore reached some type of steady state (where initial condition responses have reduced), or where the estimator has only a short interval of data and where initial conditions play a role.
Less well known is the fact that it can be used on state-dependant or linear parameter varying (LPV) systems. A state-dependant system is one where the system matrices depend upon states and the result is what appears to be a linear type of system model, but it is really nonlinear since the dependency of state matrices on the states themselves makes the system a nonlinear state equation model. It is very convenient to be able to approximate a nonlinear system in this type of form and then apply a Kalman filter, and possibly what looks like a linear control law to obtain a controller which is easy to explain and is intuitive to use.
If the system is nonlinear there is also the so-called extended Kalman filter (EKF). The EKF works very much like a linear Kalman filter in that the structure is like the plant model with a feedback loop around it. However, because the system is really nonlinear the gain has to be calculated online and this is achieved by linearizing the system about the current operating points and then solving for the gain in the usual way. The linearization is performed around the current state estimates and the current known control signal. This is very suitable when the system model is known in the form of equations and functions which are differentiable. The EKF has certainly been applied successfully in real industrial applications. However, not unexpectedly it is not quite as reliable as the standard Kalman filter used on a linear system. There can be divergence and stability problems, particularly if the EKF is used for parameter estimation in addition to state estimation.
There are of course other nonlinear filtering methods which may be used in control systems such as particle filtering, and the unscented Kalman filters, but the EKF is the simplest to understand, and this is often an important factor. The Kalman filter is probably the most valuable contribution to the toolkit of the advanced control systems engineer and its only competitor is probably predictive control. There is no competition of course for our old favourites the PID controller.
American Control Conference 2015, Chicago, Illinois
The 2015 ACC was held in July at the Parma House Hotel in Chicago, which provided an excellent venue for the event. This conference is well supported by industry and has a greater mix of industrial and academic research than the IEEE CDC Conference. The topics covered illustrates the rapid pace of change in control systems engineering away from the traditional subjects towards new and more systems oriented topics. For example, the workshop topics included MPC control of switching systems, decision making algorithms for unmanned vehicles, intelligent building systems, dynamic data driven application systems, enabling the grid of the future, next generation smart grids and taxonomies of interconnected systems.
The regular workshop sessions were equally varied and revealed new trends in the topic. There were sessions on battery health management systems for automotive vehicles, human in the loop control, agent based systems, energy management of buildings, cyber-physical systems, control of communication networks, precision mechatronics in high speed Nano positioning, biological systems, networked control systems, cooperative control of multi-agent systems, control of blood glucose, autonomy and machine intelligence, and new directions in power systems estimation, dynamics and control.
As usual various awards were presented at the conference and the Richard E Bellman Control Heritage Award went to Thomas Edgar and the very prestigious 2015 IEEE Control Systems Award to Professor Bruce Francis. There were several plenary and semi plenary lectures and that by Karl Hedrick (Berkley) on nonlinear control with automotive applications was particularly notable.
The subject of control engineering can perhaps now be better described as one of systems engineering since topics like machine learning, multi-agent systems and network control that are all much wider than simply traditional control engineering topics. The control engineer is now de facto, a systems engineer who deals with everything from a computer science to signal processing and telecommunications. This trend was clearly evident from the contents of the conference and demonstrates the healthy condition of the subject.
The event was very well managed and there were very few no shows and the venue was excellent. The organisers of the event are to be congratulated.
Follow Us on Linked In!!
Industrial Systems and Control is on linked in, please follow us here for all our up to date news, events and publications.
Book Review: Attractive Ellipsoids in Robust Control, Published by Birkhauser, £67.99
This text published by Birkhauser introduces a relatively new robust control design technique. This is applicable to a reasonably wide class of continuous time systems. In fact it covers nonlinear affine control systems in the presence of uncertainty. It provides a constructive solution that is claimed to be easily implementable. The solutions guarantee certain stability properties and even if the uncertainty description does not match the actual uncertainly such stability guarantees are comforting. The book contains mechanical and electrical mechanical system examples and maybe suitable for some advanced courses on control. It is suitable for researchers and academics in robust control.
Book Nerd.
Book Review: Linear Parameter-Varying and Time-Delay Systems, Published by: Springer, £93.50
This new text covers one of the most important modelling methods introduced over recent years in industrial applications. That is using linear parameter varying models to represent linear systems and to approximate nonlinear systems behaviour. Successful industrial applications are found in areas such as automotive engine control and wind turbine power control. The book begins with an introduction to LPV systems which include the different ways to represent LPV models. It then turns to the rather difficult problem of the stability of LPV systems and the tools that are available. Various ways in which these systems can then be controlled is then considered including robust control design methods.
In the second part of the text time-delay systems are considered. Problems with transport delays arise in all sorts of industrial applications and they are a frequent cause of poor performance. Very often controllers are retuned to allow for delays in systems which results in poor performance and even instability. There is a full chapter on the stability of time delay systems.
In the third part of the text both LPV models and time delays are considered which exacerbate the control problems involved. Both filtering and control problems are considered in this third part pf the text.
The book has an interesting layout in that important theorems are for example made to stand out by having a different background colour (grey) the material is also very nicely organised with an excellent set of references and good examples. The book is highly recommended for anyone, either student or engineer, who is likely to work on industrial applications that use such a modelling method. It is written in a very accessible form and the results are clearly presented.
Book Nerd
Contents
Hybrid Control Systems for New Challenges by Professor Mike J Grimble
ACTC News
- Advanced Control Systems, 22nd - 25th September 2015, Glasgow
- Developments in Smith Predictor Control
- Applications News
- The Flexibility of Kalman Filters
- American Control Conference 2015, Chicago, Illinoi
- Follow Us on Linked In!!
- Book Review: Attractive Ellipsoids in Robust Control, Published by: Birkhauser, £67.99
- Book Review: Linear Parameter-Varying and Time-Delay Systems, Published by: Springer, £93.50
Forthcoming ISC/ACTC Events
Early Bird Discounts Avaliable
We are offering an "Early Bird Discount" if registration is received 2 weeks before the course start date (see online registration form). Employees of ACTC member companies are entitled to two places free of charge. Note that all courses offered can also be provided at your company premises through special arrangements, please contact us for more information.
- Training Course: Advanced Control Systems , 22nd - 25th September
- Training Course: Control Fundamentals, 24-26 November 2015
Other Events of Interest
- External Event: 5th IFAC Conference on Nonlinear Model Predictive Control, 17-20 September, Seville
- External Event: 2016 American Control Conference, 6-8 July, Boston